The Revolutionary Impact of Training Data for Self-Driving Cars
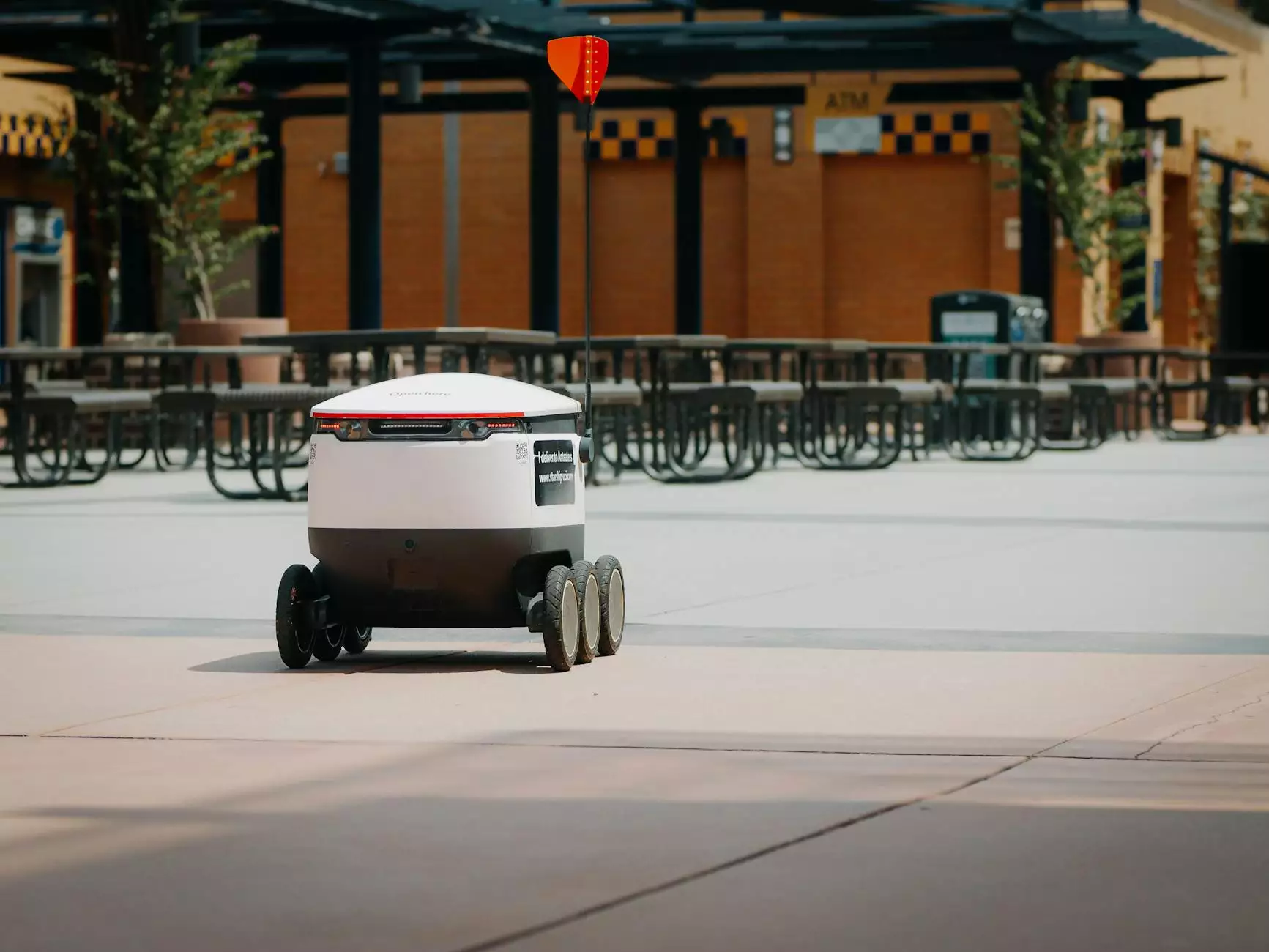
In the rapidly evolving landscape of modern transportation, the advent of self-driving cars marks a significant milestone. These vehicles are not merely a technical marvel; they symbolize a transformative shift that will redefine how we perceive mobility. At the heart of this revolution lies training data for self-driving cars, a critical component that dictates the efficiency, safety, and reliability of autonomous driving systems.
Understanding the Mechanics of Self-Driving Technology
Self-driving cars rely heavily on an intricate system of sensors, algorithms, and advanced machine learning. However, the most crucial element is undoubtedly the training data. This data is what enables the vehicle's artificial intelligence to learn from millions of real-world scenarios, ensuring it can navigate complex environments effectively.
The Importance of High-Quality Training Data
High-quality training data serves as the foundation for developing robust AI systems. In the context of self-driving cars, this data comes from various sources, including:
- Real-Time Sensor Data: Information gathered from cameras, LiDAR, radar, and ultrasonic sensors.
- Vehicle Behavior: Data on how vehicles react under different conditions, including weather, traffic, and road types.
- Cultural Context: Understanding regional driving norms and pedestrian behaviors that vary by location.
- Simulation Data: Artificial environments where potential driving scenarios can be tested safely.
Building the Future: Training Self-Driving Cars
The process of training self-driving cars involves a series of intricate steps. Developers use immense datasets to train neural networks that facilitate decision-making in real time. This training process is crucial for achieving a level of autonomy that can be deemed safe for public roads.
Steps in Training a Self-Driving Car
- Data Collection: Vast amounts of data are collected from various sources, ensuring a diverse dataset.
- Data Annotation: Human annotators label the data to indicate objects and relevant attributes, such as pedestrians, cyclists, and road signs.
- Model Training: Machine learning algorithms are trained using the annotated data to recognize patterns and predict outcomes.
- Validation: Models are tested on separate datasets to ensure accuracy and reliability.
- Real-World Testing: Finally, vehicles are tested in real-world conditions to fine-tune their responses.
The Role of Businesses Like Keymakr in This Ecosystem
With the growing reliance on innovative technologies such as self-driving cars, businesses like Keymakr can play a pivotal role. While primarily associated with home services and locksmiths, they can leverage technological advancements in various ways:
Adopting Innovative Solutions
Integration of Self-Driving Technology: As self-driving systems mature, they can enhance service delivery in several domains, including home services. Imagine autonomous vehicles equipped with locksmith tools delivering secure solutions directly to customers' doorsteps. Such scenarios exemplify how integration can enhance operational efficiencies.
Ensuring Security and Safety
As the architecture of self-driving vehicles becomes more complex, it is paramount to ensure security measures are in place. This involves:
- Regular updates and maintenance of self-driving systems.
- Implementing encryption and cybersecurity protocols to safeguard data.
- Training personnel to manage and operate such technologies effectively.
Benefits of Self-Driving Cars to the Broader Economy
As industries begin to recognize the benefits of self-driving cars, the economy stands to gain considerably. Here’s how:
- Increased Efficiency: Autonomous vehicles can optimize routes, reducing travel times and fuel consumption.
- Enhanced Safety: The potential reduction in accidents due to human error can significantly lower insurance costs.
- Job Creation: Emerging technologies will create jobs related to maintenance, programming, and oversight of autonomous systems.
- Environmental Benefits: Electric self-driving vehicles can reduce carbon footprints, contributing to environmental goals.
Challenges Ahead: Navigating the Future
Despite the promising future, the journey to widespread acceptance of self-driving cars is fraught with challenges. These include:
Regulatory Hurdles
Legal Framework: Governments must establish comprehensive regulations that govern the testing and use of autonomous vehicles on public roads. This requires collaboration between industry leaders, policymakers, and the public.
Public Perception
As with any groundbreaking technology, there’s often skepticism among consumers. Building trust in self-driving technology through transparency and proven reliability is crucial. Education about the benefits of training data for self-driving cars and its effectiveness will be essential in overcoming these hurdles.
Technological Limitations
Current technology faces challenges such as:
- Predicting unforeseen accidents and behaviors of other road users.
- Handling adverse weather conditions that can impair sensor functionality.
- Designing systems that are capable of making ethical decisions in emergency scenarios.
Conclusion: Embracing the Future of Transportation
The future of transportation is bright, thanks in large part to the advancements brought by training data for self-driving cars. This technology holds the potential to reshape not only how we drive but how businesses, including those in the home services industry like Keymakr, operate and deliver value.
As we embrace these changes, it is crucial for stakeholders across various sectors to collaborate, keeping the lines of communication open as we work together towards a safer, more efficient future.
The potential of self-driving cars extends beyond mere transportation; it embodies a holistic transformation of our societal framework. In the coming years, we are sure to witness a shift that will bring both conveniences and profound changes to our everyday lives.
training data for self driving cars